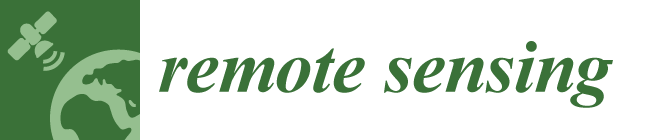
Journal Menu
► Journal MenuJournal Browser
► Journal BrowserSpecial Issue "Remote Sensing Data Application, Data Reanalysis and Advances for Mesoscale Numerical Weather Models"
A special issue of Remote Sensing (ISSN 2072-4292). This special issue belongs to the section "Atmospheric Remote Sensing".
Deadline for manuscript submissions: 25 February 2024 | Viewed by 2368
Special Issue Editors
Interests: radar data assimilation for short-term severe weather forecasting; high performance computing in data assimilation and numerical weather prediction
Interests: Doppler weather radar data assimilation; satellite remote sensing observation data assimilation; integrated variational hybrid assimilation system development; wind, solar and other renewable energy research
Special Issues, Collections and Topics in MDPI journals
Interests: satellite data assimilation; radar data assimilation; ensemble–variational data assimilation; satellite data application; numerical model prediction; severe weather simulation
Interests: global and regional reanalysis; satellite remote sensing data assimilation; coupled chemistry-meteorology data assimilation
Interests: atmospheric numerical and statistical modeling (application and development); boundary layer and turbulence; earth-atmosphere interactions; atmospheric composition; trace gas (greenhouse gas) emissions; machine learning application of atmospheric sciences
Interests: satellite remote sensing observation data assimilation; radiance data application for cloud retrievals; ensemble–variational data assimilation; radar data assimilation
Special Issues, Collections and Topics in MDPI journals
Special Issue Information
Dear Colleagues,
Recent progress in computer technology and computing capabilities has facilitated more advanced applications of remote sensing data in mesoscale numerical weather models. Furthermore, the developments of remote sensing technology continuously provide new data types. Such advances will benefit both numerical weather prediction (NWP) for severe and high-impact weather events and the quality of regional/global data reanalysis. This Special Issue seeks innovative submissions that are related to improving the accuracy of mesoscale weather models through remote sensing data assimilations, new remote sensing networks, or other remote sensing data applications that improve the prediction of high-impact weather events, air quality research, land & water monitoring, and the decision making involved in such predictions, as well as applications of and enhancements in regional or global data reanalysis with remote sensing data.
Dr. Yunheng Wang
Dr. Feifei Shen
Dr. Xin Li
Dr. Lipeng Jiang
Dr. Yuyan Cui
Dr. Dongmei Xu
Guest Editors
Manuscript Submission Information
Manuscripts should be submitted online at www.mdpi.com by registering and logging in to this website. Once you are registered, click here to go to the submission form. Manuscripts can be submitted until the deadline. All submissions that pass pre-check are peer-reviewed. Accepted papers will be published continuously in the journal (as soon as accepted) and will be listed together on the special issue website. Research articles, review articles as well as short communications are invited. For planned papers, a title and short abstract (about 100 words) can be sent to the Editorial Office for announcement on this website.
Submitted manuscripts should not have been published previously, nor be under consideration for publication elsewhere (except conference proceedings papers). All manuscripts are thoroughly refereed through a single-blind peer-review process. A guide for authors and other relevant information for submission of manuscripts is available on the Instructions for Authors page. Remote Sensing is an international peer-reviewed open access semimonthly journal published by MDPI.
Please visit the Instructions for Authors page before submitting a manuscript. The Article Processing Charge (APC) for publication in this open access journal is 2700 CHF (Swiss Francs). Submitted papers should be well formatted and use good English. Authors may use MDPI's English editing service prior to publication or during author revisions.
Keywords
- advances in remote sensing data assimilation
- new types of remote sensing observations, network design or data analysis with numerical models
- convective-allowing and/or regional numerical model developments
- probabilistic prediction methods
- verification methods and statistical modelling
- new developments in artificial intelligence for numerical models
- regional and global data reanalysis techniques
- coupled data assimilation
- air quality research