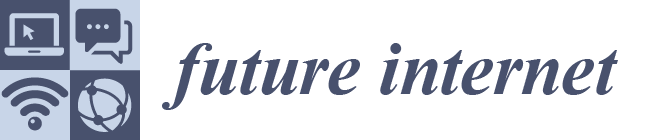
Journal Menu
► Journal MenuJournal Browser
► Journal BrowserSpecial Issue "Information and Future Internet Security, Trust and Privacy II"
A special issue of Future Internet (ISSN 1999-5903). This special issue belongs to the section "Cybersecurity".
Deadline for manuscript submissions: 10 September 2023 | Viewed by 2850
Special Issue Editors
Interests: cyber security; intrusion detection; mobile security and authentication; HCI security; malware analysis
Special Issues, Collections and Topics in MDPI journals
Interests: security in ubiquitous computing; secure collaboration in open dynamic systems; pervasive computing environments; sensor networks and the Internet of Things (IoT)
Special Issues, Collections and Topics in MDPI journals
Special Issue Information
Dear Colleagues,
Currently, the Internet of things (IoT) enables billions of Internet-connected devices, e.g., smart sensors, to communicate and interact with each other over the network/Internet worldwide. It can offer remote monitoring and control, and is being adopted in many domains. For example, it is the basis for smart cities, helping to achieve better quality of life and lower consumption of resources. In addition, smartphones are the most commonly used IoT devices, and can help control washing machines, refrigerators, or cars. However, the IoT also faces many challenges concerning information and Internet security. For example, attackers can impersonate a relay node to compromise the integrity of information during communications. When they control or infect several internal nodes in an IoT network, the security of the whole distributed environment would be greatly threatened. Hence, there is a need to safeguard information and the Internet environment against the plethora of modern external and internal threats.
This Special Issue will focus on information and Internet security in an attempt to solicit the latest technologies, solutions, case studies, and prototypes on this topic.
Dr. Weizhi Meng
Dr. Christian D. Jensen
Guest Editors
Manuscript Submission Information
Manuscripts should be submitted online at www.mdpi.com by registering and logging in to this website. Once you are registered, click here to go to the submission form. Manuscripts can be submitted until the deadline. All submissions that pass pre-check are peer-reviewed. Accepted papers will be published continuously in the journal (as soon as accepted) and will be listed together on the special issue website. Research articles, review articles as well as short communications are invited. For planned papers, a title and short abstract (about 100 words) can be sent to the Editorial Office for announcement on this website.
Submitted manuscripts should not have been published previously, nor be under consideration for publication elsewhere (except conference proceedings papers). All manuscripts are thoroughly refereed through a single-blind peer-review process. A guide for authors and other relevant information for submission of manuscripts is available on the Instructions for Authors page. Future Internet is an international peer-reviewed open access monthly journal published by MDPI.
Please visit the Instructions for Authors page before submitting a manuscript. The Article Processing Charge (APC) for publication in this open access journal is 1600 CHF (Swiss Francs). Submitted papers should be well formatted and use good English. Authors may use MDPI's English editing service prior to publication or during author revisions.
Related Special Issue
Planned Papers
The below list represents only planned manuscripts. Some of these manuscripts have not been received by the Editorial Office yet. Papers submitted to MDPI journals are subject to peer-review.
Title: IntruNet: A Hybrid Deep Learning Framework for Network Intrusion Detection
Authors: Roseline Oluwaseun Ogundokun (1); Chinecherem Umezuruike (2)
Affiliation: 1 Department of Multimedia Engineering, Kaunas University of Technology, Kaunas, Lithuania
2 Department of Software Engineering, Bowen University Iwo, Osun State, Nigeria
Abstract: Given the ever-changing nature of cyber-attacks, network security identification and prevention of network breaches offer significant difficulties. The current research introduces IntruNet, an innovative hybrid deep learning (DL) model developed for network intrusion detection (NID). IntruNet seeks to improve the reliability, effectiveness, and versatility of intrusion detection systems (IDS) by integrating the potential of multiple methodologies for DLs, such as Convolutional Neural Networks (CNN), Long Short-Term Memory (LSTM) networks, and Deep Neural Networks (DNN).
IntruNet uses the spatially distributed feature extraction (FE) powers of convolutional neural networks (CNNs) to identify tiny patterns in network connection data. LSTM models concurrently permit the simulation of time-dependent variations in network usage, which facilitates the recognition of complicated attack patterns. By incorporating each of these elements within a single structure and supplementing them with DNNs for classification, IntruNet provides an in-depth method for dependable intrusion detection.
The suggested model undergoes training and evaluation employing broad datasets that include both normal and malicious communication over the network, permitting IntruNet to acquire distinguishing characteristics and make inferences to fresh arising instances of attack. The findings from experiments indicate that IntruNet surpasses conventional IDSs, with enhanced accuracy in detecting attacks and lowered false favourable rates (FPR).
The deep hybrid learning (DL) framework of IntruNet facilitates instantaneous analysis of massive communication over the network, thus allowing the prompt identification and resolution of possible security violations. The framework's comprehensibility permits security specialists to obtain knowledge about the key characteristics that help with intrusion detection, thereby facilitating the examination and remediation of detected attacks.
The contribution made by this research is the creation of IntruNet, a hybrid DL framework that overcomes the drawbacks associated with traditional IDSs. IntruNet demonstrates the possible use of DL approaches to improve network intrusion detection powers by integrating CNN, LSTM, and DNN. The suggested model presents an exciting possibility for enhancing the security posture of network facilities, thereby minimizing the potential hazards associated with constantly evolving cyber threats.